Explore
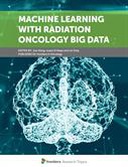
Machine Learning With Radiation Oncology Big Data
Jun Deng, Issam El Naqa, Lei Xing
2019
0 Ungluers have
Faved this Work
Login to Fave
Radiation oncology is uniquely positioned to harness the power of big data as vast amounts of data are generated at an unprecedented pace for individual patients in imaging studies and radiation treatments worldwide. The big data encountered in the radiotherapy clinic may include patient demographics stored in the electronic medical record (EMR) systems, plan settings and dose volumetric information of the tumors and normal tissues generated by treatment planning systems (TPS), anatomical and functional information from diagnostic and therapeutic imaging modalities (e.g., CT, PET, MRI and kVCBCT) stored in picture archiving and communication systems (PACS), as well as the genomics, proteomics and metabolomics information derived from blood and tissue specimens. Yet, the great potential of big data in radiation oncology has not been fully exploited for the benefits of cancer patients due to a variety of technical hurdles and hardware limitations.With recent development in computer technology, there have been increasing and promising applications of machine learning algorithms involving the big data in radiation oncology. This research topic is intended to present novel technological breakthroughs and state-of-the-art developments in machine learning and data mining in radiation oncology in recent years.
This book is included in DOAB.
Why read this book? Have your say.
You must be logged in to comment.
Rights Information
Are you the author or publisher of this work? If so, you can claim it as yours by registering as an Unglue.it rights holder.Downloads
This work has been downloaded 393 times via unglue.it ebook links.
- 104 - mobi (CC BY) at Unglue.it.
- 177 - epub (CC BY) at Unglue.it.
- 112 - pdf (CC BY) at Unglue.it.
Keywords
- artificial intelligence
- big data
- deep learning
- Machine learning
- personalized medicine
- precision medicine
- Radiation Oncology
Links
DOI: 10.3389/978-2-88945-730-4Editions
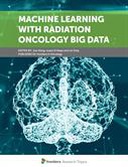