Explore
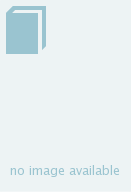
Statistical Methods for the Analysis of Genomic Data
Hui Jiang (editor) and Zhi He (editor)
2020
0 Ungluers have
Faved this Work
Login to Fave
In recent years, technological breakthroughs have greatly enhanced our ability to understand the complex world of molecular biology. Rapid developments in genomic profiling techniques, such as high-throughput sequencing, have brought new opportunities and challenges to the fields of computational biology and bioinformatics. Furthermore, by combining genomic profiling techniques with other experimental techniques, many powerful approaches (e.g., RNA-Seq, Chips-Seq, single-cell assays, and Hi-C) have been developed in order to help explore complex biological systems. As a result of the increasing availability of genomic datasets, in terms of both volume and variety, the analysis of such data has become a critical challenge as well as a topic of great interest. Therefore, statistical methods that address the problems associated with these newly developed techniques are in high demand. This book includes a number of studies that highlight the state-of-the-art statistical methods for the analysis of genomic data and explore future directions for improvement.
This book is included in DOAB.
Why read this book? Have your say.
You must be logged in to comment.
Rights Information
Are you the author or publisher of this work? If so, you can claim it as yours by registering as an Unglue.it rights holder.Downloads
This work has been downloaded 162 times via unglue.it ebook links.
- 56 - pdf (CC BY) at Unglue.it.
- 102 - pdf (CC BY) at res.mdpi.com.
Keywords
- Bayes factor
- Bayesian mixed-effect model
- boosting
- Classification
- classification boundary
- clustering analysis
- convolutional neural networks
- CpG sites
- deep learning
- DNA Methylation
- expectation-maximization algorithm
- false discovery rate control
- feed-forward neural networks
- gaussian finite mixture model
- GEE
- Gene expression
- gene regulatory network
- gene set enrichment analysis
- integrative analysis
- kernel method
- lipid–environment interaction
- longitudinal lipidomics study
- Machine learning
- Mathematics & science
- multiple cancer types
- n/a
- network substructure
- nonparanormal graphical model
- OMICS data
- Ordinal responses
- penalized variable selection
- prognosis modeling
- Reference, information & interdisciplinary subjects
- Research & information: general
- RNA-Seq
- uncertainty
Links
DOI: 10.3390/books978-3-03936-141-0Editions
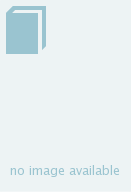