Explore
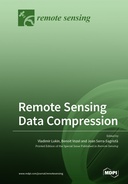
Remote Sensing Data Compression
0 Ungluers have
Faved this Work
Login to Fave
A huge amount of data is acquired nowadays by different remote sensing systems installed on satellites, aircrafts, and UAV. The acquired data then have to be transferred to image processing centres, stored and/or delivered to customers. In restricted scenarios, data compression is strongly desired or necessary. A wide diversity of coding methods can be used, depending on the requirements and their priority. In addition, the types and properties of images differ a lot, thus, practical implementation aspects have to be taken into account. The Special Issue paper collection taken as basis of this book touches on all of the aforementioned items to some degree, giving the reader an opportunity to learn about recent developments and research directions in the field of image compression. In particular, lossless and near-lossless compression of multi- and hyperspectral images still remains current, since such images constitute data arrays that are of extremely large size with rich information that can be retrieved from them for various applications. Another important aspect is the impact of lossless compression on image classification and segmentation, where a reasonable compromise between the characteristics of compression and the final tasks of data processing has to be achieved. The problems of data transition from UAV-based acquisition platforms, as well as the use of FPGA and neural networks, have become very important. Finally, attempts to apply compressive sensing approaches in remote sensing image processing with positive outcomes are observed. We hope that readers will find our book useful and interesting
This book is included in DOAB.
Why read this book? Have your say.
You must be logged in to comment.
Rights Information
Are you the author or publisher of this work? If so, you can claim it as yours by registering as an Unglue.it rights holder.Downloads
This work has been downloaded 75 times via unglue.it ebook links.
- 75 - pdf (CC BY) at Unglue.it.
Keywords
- 3D-CALIC
- CCSDS
- CCSDS 123.0-B-2
- compact data structure
- complexity
- compressed sensing
- compression impact
- compressive sensing
- Computational complexity
- coupled dictionary
- crop classification
- DACs
- data compression
- EBCOT
- Elias codes
- FAPEC
- FPGA
- fully convolutional network
- GPU
- graph filterbanks
- graph signal processing
- group convolution
- HEVC
- high bit-depth compression
- hyperspectral
- hyperspectral image
- hyperspectral image coding
- hyperspectral images
- hyperspectral imaging
- hyperspectral scenes
- image classification
- image quality
- integer-to-integer transforms
- intra coding
- invertible projection
- JPEG 2000
- JPEG2000
- k2-raster
- k2-tree
- Landsat-8
- learned compression
- lossless compression
- lossy compression
- M-CALIC
- multispectral
- multispectral image compression
- multispectral satellite images
- near-lossless hyperspectral image compression
- neural networks
- on board compression
- on-board compression
- on-board data compression
- on-board processing
- parallel computing
- partitioned extraction
- PCA
- PForDelta
- quadtree
- rate-distortion
- real time
- real-time compression
- real-time performance
- real-time transmission
- Remote sensing
- remote sensing data compression
- Rice codes
- semantic segmentation
- Sentinel-2
- Simple16
- Simple9
- singular value
- spectral image
- spectral–spatial feature
- synthetic aperture sonar
- task-driven learning
- Technology, engineering, agriculture
- Technology: general issues
- tensor decomposition
- transform
- transform coding
- UAV
- UAVs
- underwater sonar imaging
- variational autoencoder
- visual quality metrics
Links
DOI: 10.3390/books978-3-0365-2304-0Editions
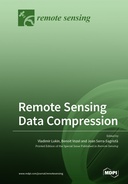