Explore
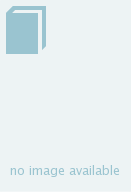
Advanced Methods of Power Load Forecasting
0 Ungluers have
Faved this Work
Login to Fave
This reprint introduces advanced prediction models focused on power load forecasting. Models based on artificial intelligence and more traditional approaches are shown, demonstrating the real possibilities of use to improve prediction in this field. Models of LSTM neural networks, LSTM networks with a SESDA architecture, in even LSTM-CNN are used. On the other hand, multiple seasonal Holt-Winters models with discrete seasonality and the application of the Prophet method to demand forecasting are presented. These models are applied in different circumstances and show highly positive results. This reprint is intended for both researchers related to energy management and those related to forecasting, especially power load.
This book is included in DOAB.
Why read this book? Have your say.
You must be logged in to comment.
Rights Information
Are you the author or publisher of this work? If so, you can claim it as yours by registering as an Unglue.it rights holder.Downloads
This work has been downloaded 80 times via unglue.it ebook links.
- 80 - pdf (CC BY) at Unglue.it.
Keywords
- artificial neural network
- Attention
- bidirectional long short-term memory
- CNN
- deep learning
- deep neural network
- demand
- DIMS
- encoder decoder
- forecast
- galvanizing
- Holt–Winters model
- irregular
- load
- long-term forecasting
- LSTM
- Machine learning
- Mathematics & science
- multi-layer stacked
- multiple seasonality
- Neural Network
- online training
- parameters tuning
- peak load
- Physics
- power system
- Prophet model
- recurrent neural network
- Reference, information & interdisciplinary subjects
- Research & information: general
- short-term electrical load forecasting
- short-term load forecast
- short-term load forecasting
- statistical analysis
- time series
Links
DOI: 10.3390/books978-3-0365-4217-1Editions
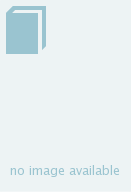