Explore
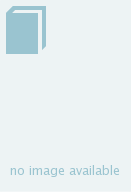
Deep Learning and Computer Vision in Remote Sensing-II
0 Ungluers have
Faved this Work
Login to Fave
Computer Vision (CV) have seen a massive rise in popularity in the remote sensing field over the last few years. This success is mostly due to the effectiveness of deep learning (DL) algorithms. However, remote sensing data acquisition and annotation, as well as information extraction from massive remote sensing data, are still challenging. This reprint collected novel developments in the field of deep learning and computer vision methods for remote sensing. Papers dealing with fundamental theoretical analyses, as well as those demonstrating their application to real-world problems, have been published. With practical examples and real-world case studies, this reprint provides a valuable resource for researchers, professionals, and students seeking to harness the power of deep learning in the field of remote sensing. Here are some major topics that are addressed in this reprint: Satellite image processing and analysis based on deep learning; Deep learning for object detection, image classification, and semantic and instance segmentation; Deep learning for remote sensing scene understanding and classification; Transfer learning, deep reinforcement learning for remote sensing; Supervised and unsupervised representation learning for remote sensing environments.
This book is included in DOAB.
Why read this book? Have your say.
You must be logged in to comment.
Rights Information
Are you the author or publisher of this work? If so, you can claim it as yours by registering as an Unglue.it rights holder.Downloads
This work has been downloaded 51 times via unglue.it ebook links.
- 51 - pdf (CC BY) at Unglue.it.
Keywords
- 1D landmark representation
- artificial intelligence
- attention mechanism
- automatic target recognition
- building extraction
- complex-valued capsule network
- complex-valued U-Net
- Computer science
- Computing & information technology
- convolutional neural network (CNN)
- convolutional neural networks
- convolutional neural networks (CNNs)
- data augmentation
- deep learning
- deep supervision
- dynamic resolution adaptation
- earth observation
- Economics, finance, business & management
- few-shot feature adaptation
- generative family
- grassland grazing livestock
- HRNet
- Industry & industrial studies
- Information technology industries
- knowledge inference module
- land use and land cover classification
- landmark regression
- Landsat
- lightweight model
- loss function
- Machine learning
- Media, information & communication industries
- multi-branch convolution
- multi-feature fusion and attention network
- multi-label classification
- multi-scale object detection
- neural architecture search
- neural processes
- object detection
- polarimetric synthetic aperture radar
- pose estimation
- Remote sensing
- remote sensing image
- remote sensing imagery
- remote sensing images
- remote-sensing image
- semantic segmentation
- small-object detection
- space target
- spacecraft recognition
- sparse regularization
- synthetic aperture radar
- thema EDItEUR::U Computing and Information Technology::UY Computer science
- transfer learning
- transformer
- tree ensemble methods
- unmanned aerial vehicle (UAV)
Links
DOI: 10.3390/books978-3-0365-9365-4Editions
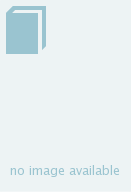