Explore
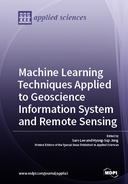
Machine Learning Techniques Applied to Geoscience Information System and Remote Sensing
Hyung-Sup Jung and Saro Lee
2019
0 Ungluers have
Faved this Work
Login to Fave
As computer and space technologies have been developed, geoscience information systems (GIS) and remote sensing (RS) technologies, which deal with the geospatial information, have been rapidly maturing. Moreover, over the last few decades, machine learning techniques including artificial neural network (ANN), deep learning, decision tree, and support vector machine (SVM) have been successfully applied to geospatial science and engineering research fields. The machine learning techniques have been widely applied to GIS and RS research fields and have recently produced valuable results in the areas of geoscience, environment, natural hazards, and natural resources. This book is a collection representing novel contributions detailing machine learning techniques as applied to geoscience information systems and remote sensing.
This book is included in DOAB.
Why read this book? Have your say.
You must be logged in to comment.
Rights Information
Are you the author or publisher of this work? If so, you can claim it as yours by registering as an Unglue.it rights holder.Downloads
This work has been downloaded 249 times via unglue.it ebook links.
- 25 - pdf (CC BY-NC-ND) at Unglue.it.
Keywords
- ALS point cloud
- alternating direction method of multipliers
- artificial neural network
- bagging ensemble
- Bayes net
- boosted regression tree
- change detection
- Classification
- classification-based learning
- coarse particle
- Colorization
- constrained spatial smoothing
- convergence time
- convolutional network
- convolutional neural networks
- crop
- data mining techniques
- deep learning
- environmental variables
- feature selection
- Gaofen-2
- geoherb
- GIS
- gray-level co-occurrence matrix
- grayscale aerial image
- gully erosion
- high-resolution
- hybrid model
- hybrid structure convolutional neural networks
- information gain
- ionospheric delay constraints
- land subsidence
- land use/land cover (LULC)
- landsat image
- landslide
- landslide prediction
- landslide susceptibility
- landslide susceptibility map
- large scene
- leaf area index (LAI)
- logistic
- Logistic Model Trees
- logistic regression
- logit boost
- Machine learning
- mapping
- materia medica resource
- model assessment
- model switching
- model validation
- multi-scale
- multilayer perceptron
- n/a
- naïve Bayes
- neural networks
- object detection
- one-class classifiers
- optical remote sensing
- Panax notoginseng
- panchromatic
- particulate matter 10 (PM10)
- precise weighting
- predictive accuracy
- Qaidam Basin
- random forest
- random forest regression
- real-time precise point positioning
- Remote sensing
- remote sensing image segmentation
- reproducible research
- SCAI
- sensitivity analysis
- Sentinel-2
- single-class data descriptors
- spatial predictions
- spatial predictive models
- spatial sparse recovery
- spatial spline regression
- spectral bands
- support vector machine
- Texture
- traffic CO
- traffic CO prediction
- training sample size
- transfer learning
- TRMM
- unmanned aerial vehicle
- variable selection
- Vietnam
- weights of evidence
- winter wheat spatial distribution
Links
DOI: 10.3390/books978-3-03921-216-3Editions
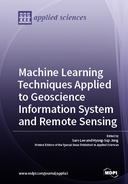
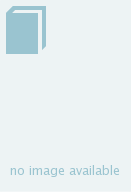